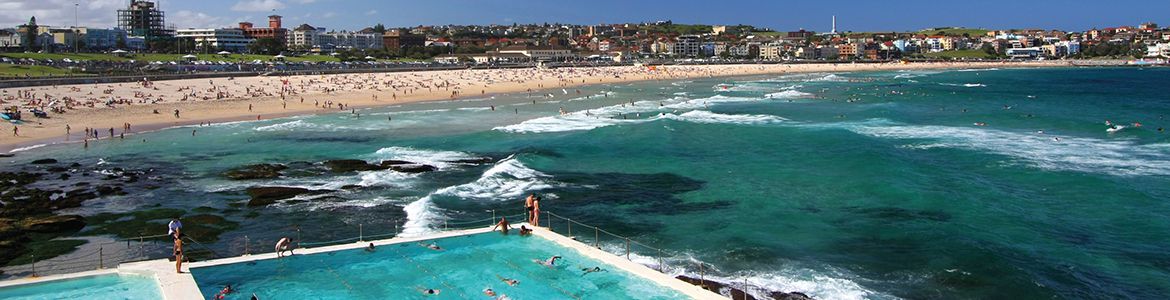
ECR/PhD Workshop Speakers
Keynote
Prof. Beng Chin OOI, National University of Singapore
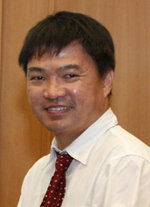
Bio: Beng Chin is a Distinguished Professor of Computer Science, NGS faculty member and Director of Smart Systems Institute (SSI@NUS) at the National University of Singapore (NUS), an adjunct Chang Jiang Professor at Zhejiang University, China, and the director of NUS AI Innovation and Commercialization Centre at Suzhou, China. He obtained his BSc (1st Class Honors) and PhD from Monash University, Australia, in 1985 and 1989 respectively. Beng Chin's research interests include database systems, distributed and blockchain systems, and large scale analytics, in the aspects of system architectures, performance issues, security, accuracy and correctness. He works closely with the industry (eg. NUHS, Jurong Health, Tan Tok Seng Hospital, Singapore General Hospital, KK Hospital on healthcare analytics and prediebetes prevention), and exploits IT for efficiency in various appplication domains, including healthcare, finance and smart city. He is a co-founder of yzBigData(2012) for Big Data Management and analytics, and Shentilium Technologies(2016) for AI- and data-driven Financial data analytics, MediLot Technologies(2018) for blockchain based healthcare data management and analytics, an advisor of a RegTech company, Cynopsis Solutions, and an advisor to blockchain based KYC traceto.io ICO. Beng Chin serves as a non-executive and independent director of ComfortDelgro, a transportation company, and a member of Hangzhou Government AI Development Committee (AI TOP 30). Beng Chin is a fellow of the ACM, IEEE, and Singapore National Academy of Science (SNAS).
Title: Translational Healthcare Research from System and Data Perspectives
Abstract: While AI and data-driven approaches are still evolving, they are likely to surpass current medical practices in the healthcare domain soon. The potential advantages are not only faster and more accurate analysis, but also the democratization of healthcare services. Notwithstanding, there are some common challenges when applying existing approaches onto the healthcare domain, due to the noise and bias of electronic health records (EHR), complex and heterogeneous feature relations, access control and data privacy and etc. In this talk, I shall discuss our design and implementation strategies: solve common challenges, instill domain knowledge, automate knowledge extraction, and enable system-based global optimization. I discuss our rationale on building a general analytics stack instead of solving individual problems, and explain how these challenges are being addressed. Several detailed technologies from both system and algorithm perspectives in our healthcare data management and analytics framework are also described. I shall also discuss our new translational project on reducing 3H (hyperglycemia, hypertension, hyperlipidemia) problems.
Invited Talks
Dr. Zhifeng Bao, RMIT
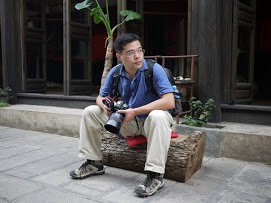
Bio: Dr Zhifeng Bao is a senior lecturer in Computer Science at RMIT University and an Honorary Fellow at The University of Melbourne, Australia. He is now the Head of RMIT’s Big Data and Database Group and the program manager of RMIT Master of Data Science Program. He received his PhD in Computer Science from National University of Singapore (NUS) in 2011. His research interests include data visualization, spatial data analytics and data cleaning, and he regularly published in top venues of Databases and Information Retrieval such as SIGMOD, SIGIR and SIGKDD. He served the Chair of WSDM19 Cup, DASFAA17 (workshop track), ER18 (demo track), and the PC member of top conferences such as VLDB, SIGMOD, SIGIR and ICDE. Zhifeng has received four best paper awards, as well as five best paper award nominations such as SIGKDD 2018 and ICDE 2009.
Title: An Exploration of Geospatial Data
Abstract: In this talk I will present, from the perspective of problem, our recent work on cleaning, searching, visualizing various forms of geospatial data, such as point of interest data, area of interest data and trajectory data. From the perspective of methodology, I will present indexing techniques, pruning paradigms, and theoretical analysis of algorithms. At the end of the talk several system prototypes of geospatial data exploration will be demonstrated to the audience.
Dr. Renata Borovica-Gajic, University of Melbourne
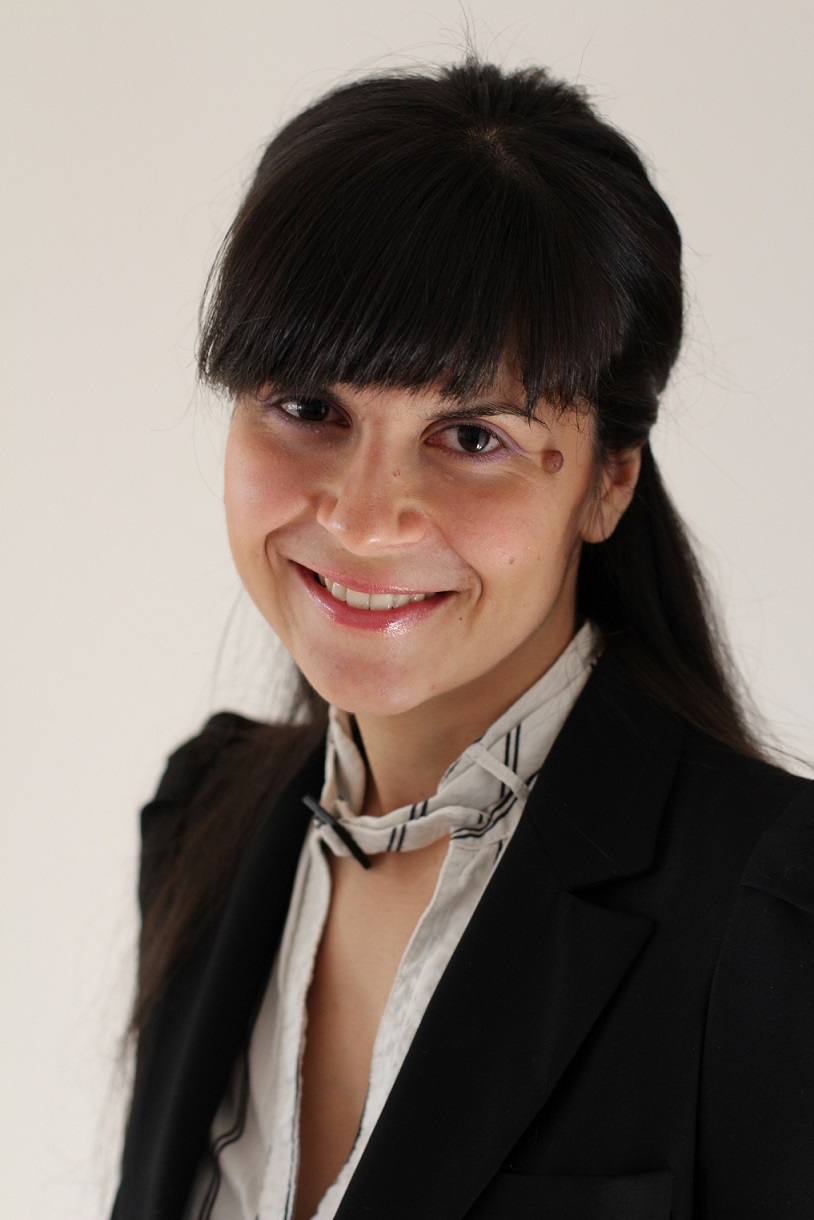
Bio: Renata Borovica-Gajic holds a position of Lecturer in Data Analytics in the School of Computing and Information Systems at The University of Melbourne. Dr Borovica-Gajic received her Ph.D. degree in Computer Science from Swiss Federal Institute of Technology in Lausanne (EPFL), Switzerland in 2016. Renata's research focuses on solving data management problems when storing, accessing and processing massive data sets, enabling faster, more predictable, and cheaper data analysis as a result. She envisions database systems as dynamic entities able to adjust query processing strategies to fit the characteristics of data and usage patterns. She is also interested in the topics of scientific data management, data exploration, query optimization, physical database design, and hardware-software co-design. Her work has appeared in the premier data management conferences such as SIGMOD, VLDB, and ICDE.
Title: A Tale of Learning Databases
Abstract: The ability to perform timely, predictable and cost-effective analytical processing of large data sets to extract deep insights is a key ingredient to the success of many industrial and government domains (e.g., internet search, marketing firms, telecommunications, and healthcare). Traditional database management systems (DBMS) are, however, not the first choice for servicing these modern applications, due to long preparation steps required to set up the DBMS for analysis. In this talk, I am going to show that the game is not lost for traditional DBMSs. I will demonstrate that traditional DBMSs can still provide timely, predictable, and cost-effective analytics and join the race of servicing modern applications, if they embrace an adaptive and agile approach in which the query processing strategy is learned on the go and adjusted during query processing to fit the characteristics of: i) the user query requests (workload), ii) the data, and iii) the underlying hardware. In particular, workload-driven learning is introduced as a means of enabling efficient data exploration and reducing the time to first insight. Data-driven learning alleviates suboptimal query processing strategies by automatically transforming the access strategy during query processing to fit the observed characteristics of the data. Finally, hardware-driven learning is an example of the adaptation of the DBMS engine to the properties of new hardware technology (cold storage) as a cost-effective solution for storing the ever-increasing customer data base.
Dr. Xin Cao, The University of New South Wales

Bio: Dr. Xin Cao is currently a lecturer and an ARC DECRA Fellow (Australia Discovery Early Career Researcher Award) in School of Computer Science and Engineering at University of New South Wales (UNSW) in Australia. He received his PhD degree from Nanyang Technological University (NTU) in 2014, and received his bachelor and master degrees in computer science and technology from Zhejiang University in 2006 and 2008, respectively. Prior to studying at NTU, he worked as a scientific assistant in the Center for Data-Intensive Systems (Daisy) in Aalborg University (AAU) for two years. His research interests include data management (in particular on spatial, temporal, textual, and graph data), databases, and data mining. Most of his work was published in premier database conferences and journals, such as SIGMOD, VLDB, ICDE, VLDBJ, and TODS.
Title: Group Search in Location-Aware Social Networks
Abstract: The online social networks have greatly changed the way that people interact with each other and also with the world. With the proliferation of wireless communication techniques and GPS-equipped mobile devices (e.g., smart phones), nowadays people are able to access the social networks almost anywhere at anytime. The location information plays an important role in social network applications, because the geographical information brings social networks from virtual to reality, bridging the gap between the physical world and online social networking services. We will discuss how to efficiently search a group of objects (e.g., a community, a region, and a route etc) in such location-aware social networks.
Dr. Lijun Chang, The University of Sydney
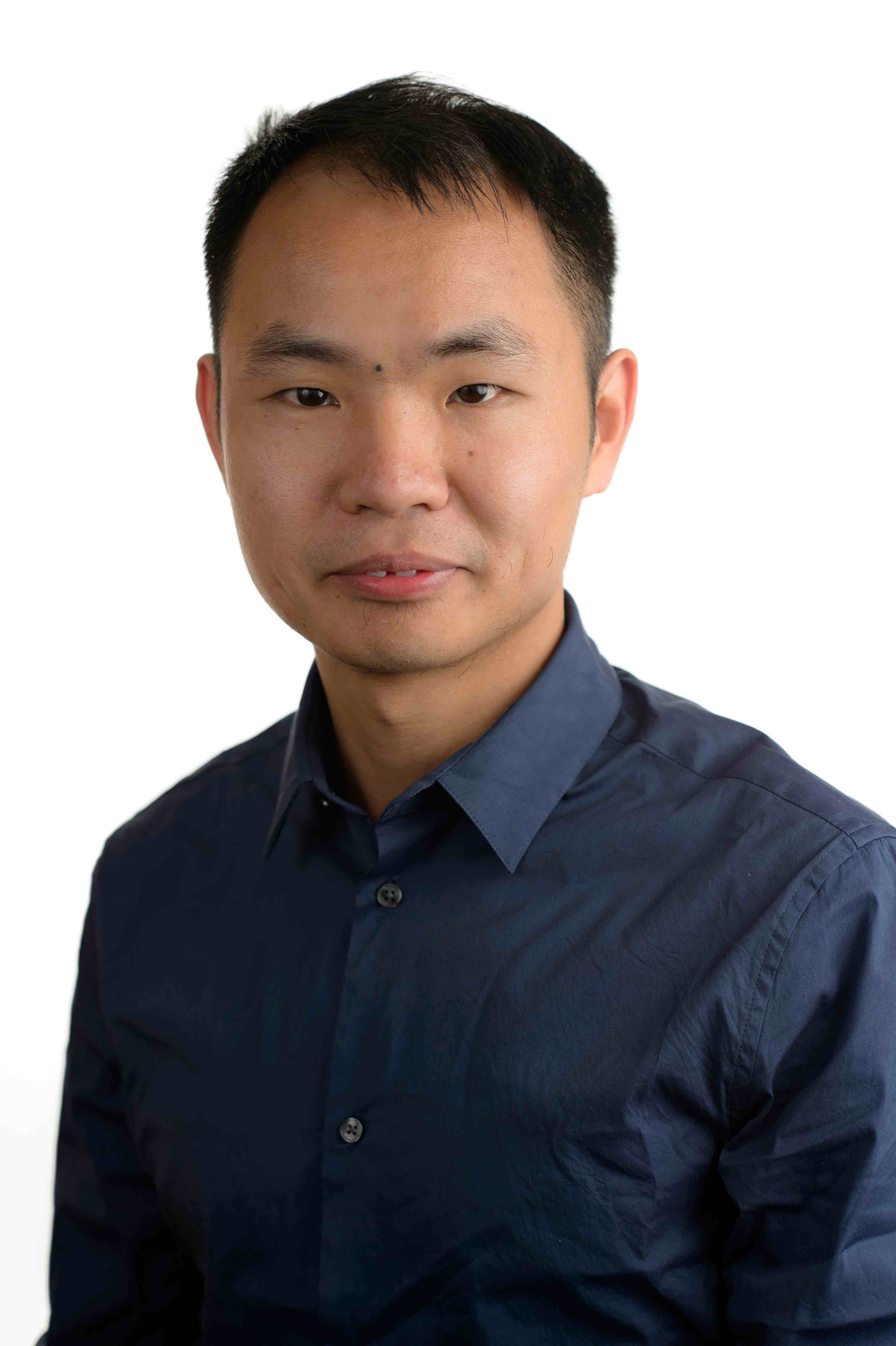
Bio: Dr. Lijun Chang is a Senior Lecturer at The University of Sydney. He received Bachelor degree from the Department of Computer Science and Technology at Renmin University of China in 2007, and Ph.D. degree from Department of Systems Engineering and Engineering Management at The Chinese University of Hong Kong in 2011. He worked as a Postdoc and then DECRA research fellow at the University of New South Wales from 2012 to 2017. His research interests are in the fields of big graph (network) analytics, with a focus on designing practical algorithms and developing theoretical foundations for massive graph analysis.
Title: Cohesive Subgraph Computation over Large Sparse Graphs
Abstract: With the rapid development of information technology, huge volumes of graph data are accumulated. An availability of rich graph data not only brings great opportunities for realizing big values of data to serve key applications, but also brings great challenges in computation. Real graphs are sparsely connected from a global point of view, but they usually contain subgraphs that are locally densely connected. Computing cohesive/dense subgraphs can either be the main goal of a graph analysis task, or act as a preprocessing step aiming to reduce/trim the graph by removing sparse/unimportant parts such that more complex and time-consuming analysis can be conducted. In this talk, I will give an introduction to the models and algorithms for the problem of cohesive subgraph computation.
A/Prof. Jianxin Li, Deakin University
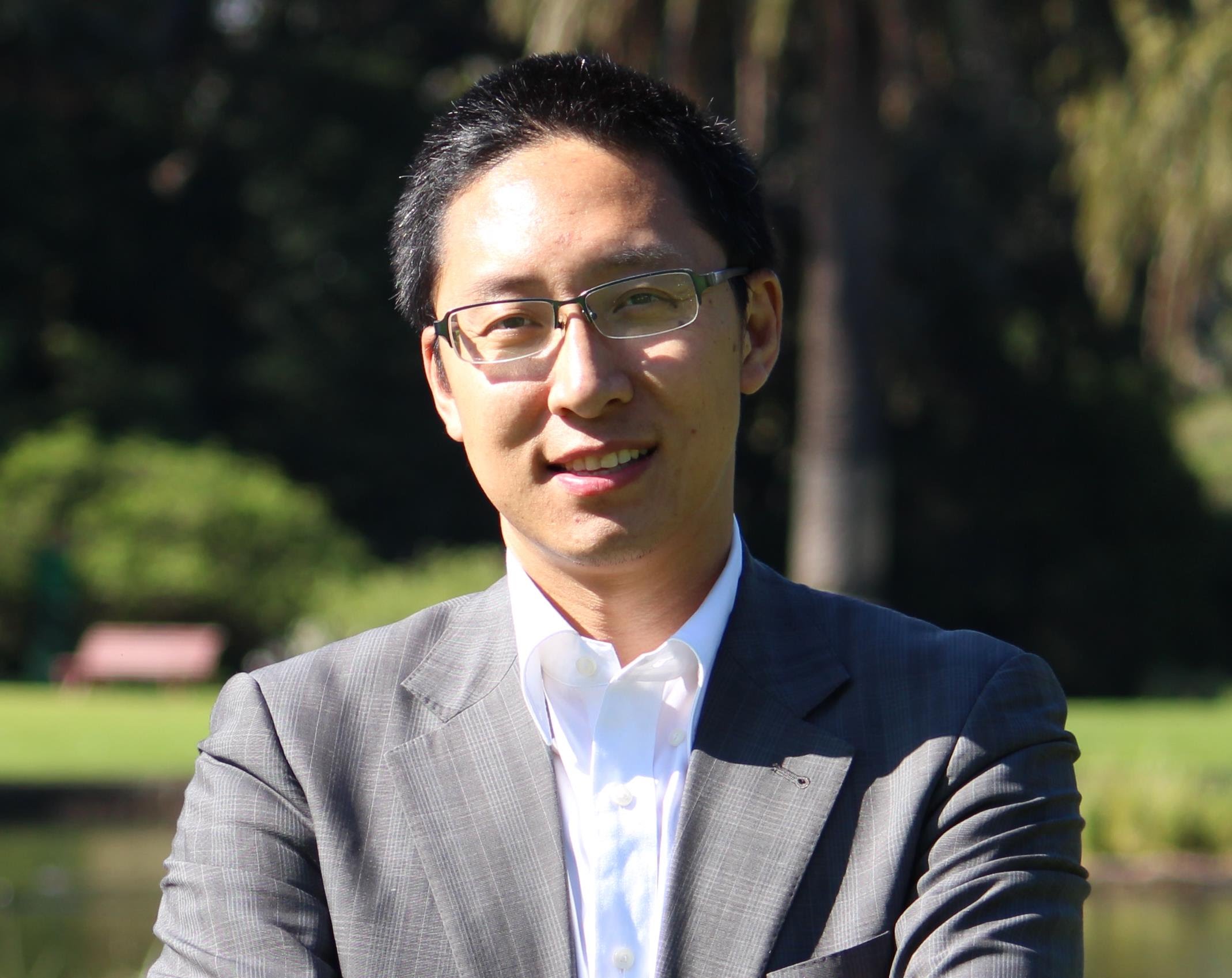
Bio: Dr Jianxin Li is an A/Professor in the School of IT, Deakin University. His research interests include social computing, query processing and optimization, and big data analytics. He has published 70 high quality research papers in top international conferences and journals, including PVLDB, IEEE ICDE, ACM WWW, IEEE ICDM, EDBT, ACM CIKM, IEEE TKDE, and ACM WWW. His professional service can be identified by different roles in academic committees, e.g., the technical program committee members in ACM SIGMOD, PVLDB, AAAI, PAKDD, IEEE ICDM, and ACM CIKM; the journal reviewer in IEEE TKDE, ACM TKDD, WWW Journal and VLDB Journal; the proceeding chairs in DASFAA 2018, ADMA 2016 and ADC 2015; and the program committee chair in the International Workshop on Social Computing 2017 and 2018; the tutorial chair in the 26th International Conference on WWW 2017; and the guest editors in international journals, such as Computational Intelligence, IET Intelligent Transport Systems, Complexity, Data Science and Engineering.
Title: Advanced Social Influence Maximization Computing over Large Social Networks
Abstract: Social media has become an emerging platform for organizations to broadcast their policies, for companies to advertise their products, and for people to propagate their opinions. In the social media data analytics, one of the most significant problems is the influence maximization problem. Given a social network and a campaign budget, the goal of influence maximization problem is to identify a set of influential users that are most likely to influence the maximum number of users in the social network. Meanwhile, the selected user set size is limited to the specified campaign budget. Thus, the small set of selected users can help campaign organizers to improve their marketing, branding, and product adoption in a profitable way. At this seminar, Jianxin will first introduce some background knowledge about social influence and the traditional influence maximization problem, and then give an overview about the recent research on the problem from different perspectives – topic-aware, location-aware, community-aware and target-aware. The presenter will mainly explain the motivation of such novel problems, the new insights, the procedure of defining these problems in an easy way, the optimization techniques in solving such problems, and their experimental evaluations. The main goal in presenting this seminar is to help audiences to know and understand the different applications of social influence in need, how the influence models are devised, the existing research challenges and the state-of-the-arts in this topic. This presentation is suitable to a broad audience who have interest in data science.
Prof. Wei Wang, The University of New South Wales

Bio: Dr. Wei Wang is a Professor at the School of Computer Science and Engineering at University of New South Wales, Australia. He received his Ph.D. degree in Computer Science from Hong Kong University of Science and Technology in 2004. His research interests include similarity search, knowledge graphs and NLP, security issues for AI models, and integration of Database and AI technologies. He has published over 100 research papers in these areas, with a majority of them in prestigious international journal (ACM TODS, VLDB Journal, IEEE TKDE) and conferences (SIGMOD, VLDB, ICDE, WWW, IJCAI, AAAI, ACL).
Title: High-dimensional Data --- At the intersection of Databases and Machine/Deep Learning
Abstract: Database and machine/deep learning have been largely developed in their own tracks. Recently, there is a trend to integrate the two areas. In this talk, we look at this integration from the viewpoint of high-dimensional data (aka high-dimensional vectors). Specifically, we discuss three topics: approximate nearest neighbour queries, adversarial examples, and estimation problems. We conclude the talk by presenting a few open challenges.